The Arc Prize Foundation, a nonprofit co-founded by AI researcher François Chollet, has launched ARC-AGI-2, a new set of tests designed to assess AI models in a unique way. And that’s what makes these tests remarkable.
How close is AGI? While other benchmarks measure how well AI systems solve mathematical or programming problems, the tests designed by Chollet and his team aim to evaluate how close artificial general intelligence (AGI) really is. To do this, the Arc Prize Foundation uses tests that assess the perceptual capacity of AI models. That’s where an “old” paradox comes into play.
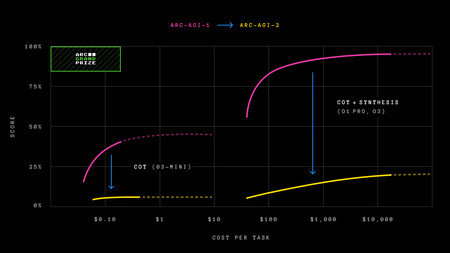
Moravec’s paradox. In 1988, Austrian engineer Hans Moravec formulated the paradox that bears his name: AI systems can make difficult tasks easy but struggle with what comes naturally to humans. You can test this by asking a generative AI model like ChatGPT, Claude, DeepSeek, and Gemini to count the letter “R” in a sentence—it will likely struggle.
What does ARC-AGI measure? ARC-AGI tests measure AI models’ ability to generalize, learn, and adapt to entirely new problems with unknown answers. Much of the focus is on abstract reasoning with visual puzzles, where AI models must identify patterns in colored grids and generate solutions accordingly. These tests reveal whether an AI model can solve problems it wasn’t trained for and whether it can generalize by extracting underlying rules from simple examples and applying them to new situations.
Memorization isn’t enough. In many benchmarks, AI systems benefit from “knowing the whole curriculum by heart” and simply apply memorized answers. Here, the goal is to reason and extrapolate like a human. The focus is on assessing whether AI models are approaching AGI—if they are, they should be able to reason like humans. However, passing this test doesn’t necessarily mean an AI system has achieved AGI.
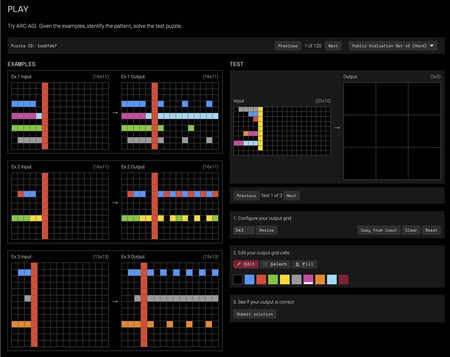
ARC-AGI-1 was useful—for a while. In early 2024, AI models struggled with the first version of ARC-AGI. By the end of the year, reasoning models emerged, and things got interesting. OpenAI’s o1-mini model passed 7.8% of the tests, while o3-low reached 76%. O3-high, though expensive, achieved 87.5%. With AI models on the verge of surpassing the benchmark, the tests needed a revision.
ARC-AGI-2 raises the bar. The new version consists of similar puzzle-like problems that require AI models to recognize visual patterns. Reasoning models like o1-pro and DeepSeek R1 barely exceed 1.3% in ARC-AGI-2, while non-reasoning models (GPT-4.5, Claude 3.7, Gemini 2.0 Flash) don’t surpass 1%. O3-low, which achieved nearly 76% in ARC-AGI-1, now reaches only 4% in ARC-AGI-2—at a cost of $200 per task. Meanwhile, humans average 60% accuracy, far surpassing current AI models. These unique puzzles, available on the project’s website, will challenge even the most curious minds.
No more brute force. The researchers behind this benchmark explain that AI models can no longer use brute force to find solutions, an issue in ARC-AGI-1. To counter this, the new efficiency metric requires models to interpret patterns in real time, “perceiving” rather than relying on stored data.
AI models need to work harder. Mike Knoop, one of the test developers, described ARC-AGI-2 as “the only unbeaten benchmark (we’re aware of) that remains easy for humans but now even harder for AI.” The results show that AI models struggle to assign semantics to puzzles—something humans do intuitively—and fail when multiple rules must be applied simultaneously to solve a problem.
Pros and cons of ARC-AGI. These tests take a unique approach, evaluating an aspect of AI models that other benchmarks overlook. However, there’s a risk: AI developers may optimize models specifically for these types of puzzles, while the models still struggle with broader generalization. Even so, ARC-AGI influences the development of AI models in interesting ways. Instead of creating “know-it-all” AI, users want systems that can reason, adapt, and tackle novel challenges.
AI models should ask new questions, not just answer old ones. A key dilemma in AI research is that while models can provide answers to known problems, they rarely ask groundbreaking questions. Critics argue that AI models don’t generate new knowledge or discover groundbreaking technologies—they merely recombine existing data to push the boundaries. While this assists human researchers, it’s still far from the AI revolution many envision.
Image | Olav Ahrens Røtne (Unsplash)
Log in to leave a comment