AI systems have no idea what they’re saying or why they’re saying it. Almost everything makes sense when they respond to users—even their mistakes. But machines don’t understand what they’re doing, they just do it. Users don’t yet understand how they think inside, but that may soon change.
Opening the black box. Researchers at Anthropic, the company that created the Claude chatbot, claim to have made an important discovery that will allow users to understand how large language models (LLMs) work. These models work like big black boxes: Users know from the start what to give them (a prompt) and what they get as a result. What remains a mystery, however, is what goes on inside these “black boxes” and how the models generate the content they do.
Why it’s essential to know how AI models “think.” AI models’ inscrutability creates significant problems. For example, it’s difficult to predict whether they will “hallucinate” or make mistakes and why they made them. Knowing exactly how they work inside would help developers better understand these errors, correct these problems, and improve their behavior.
Safer, more reliable. Knowing why AI models do what they do would also be crucial for trusting them more. These models would offer many more guarantees in areas such as privacy and data protection, which often bar companies from using them.
What about reasoning models? The emergence of models such as o1 or DeepSeek R1 has made it possible for these “reasoning” processes to appear and show their actions at any moment. The list of mini-tasks they perform (“searching the web,” “analyzing information,” etc.) is helpful. Still, the so-called “chain of reasoning” doesn’t reflect how these models process users’ requests.
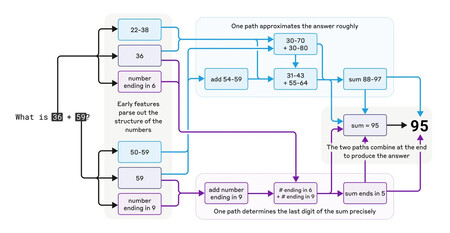
Deciphering how AI models think. Anthropic experts have created a tool that attempts to decipher this black box. It’s like MRI scans of the human brain, revealing which brain regions play a role in specific cognitive domains.
Long-term responses. Although models like Claude are trained to predict the next word in a sentence, in some tasks this LLM seems to plan longer term. For example, if you ask it to write a poem, Claude first finds words that fit the poem’s theme and then creates the phrases that generate the lines and rhymes.
One language to think in, many to translate. Although Claude supports multiple languages, Anthropic’s experts reveal that it doesn’t “think” directly in those languages. Instead, it uses concepts common to various languages to appear to think in one language before translating the output into the desired language.
Models cheat. Research shows that models can lie about what they are doing—even pretend to think when they already have the answer to your query. Joshua Batson, one of Claude’s developers, explained, “Even though it does claim to have run a calculation, our interpretability techniques reveal no evidence at all of this having occurred.”
How Anthropic’s decryption works. Anthropic’s method uses a cross-layer transcoder that analyzes sets of interpretable features rather than trying to interpret individual “neurons.” These features could be, for example, all the conjugations of a particular verb. This approach lets researchers identify entire “circuits” of neurons that tend to connect in these processes.
A good start. OpenAI tried to figure out how its AI models think in the past but didn’t succeed. Anthropic’s work has notable limitations. For example, it does’'t explain why LLMs pay more attention to certain parts of the prompt than others. Still, Batson said, “I think in another year or two, we’re going to know more about how these models think than we do about how people think.”
Images | Anthropic
Related | Anthropic May Have the Best Generative AI Product, But Even That Doesn’t Guarantee Its Survival
Log in to leave a comment