In 2013, hardly anyone had heard of DeepMind, a small AI startup. Its researchers devised the idea of making their AI system learn how to play (and win) video games. They trained it on some old Atari console titles.
Among them was Breakout. A video shows that after 10 minutes of play, the machine could barely understand anything. After two hours, it played like an expert.
After four hours, something amazing happened: The machine discovered a trick to maximize efficiency. It made the ball create a tunnel at the end, then put the ball through so it would not stop bouncing, clearing almost the entire level effortlessly.
Since then, using video games to train AI models or test their ability to adapt and complete tasks has become commonplace in the industry. This is exactly what Anthropic tried when it released Claude 3.7 Sonnet a few weeks ago.
This hybrid AI model has proven to be a remarkable breakthrough in areas such as programming and reasoning, but Anthropic wanted to test it with a unique challenge: playing the Pokémon video game.
Claude Gets Stuck
In this experiment, Anthropic’s managers wanted to see if AI systems could overcome challenges with increasingly complex skills, no longer just through training but through generalized reasoning.
Previous versions of Claude struggled even to get off the game’s home screen, but Claude 3.7 Sonnet’s “extended thinking” allows the model to “plan ahead,” remember its goals, and adapt when initial strategies fail in ways its predecessors couldn’t.
For Anthropic researchers, these improvements will ultimately help solve real-world problems. This is evident in the ARC-AGI 2 benchmark, designed to measure AI’s ability to tasks that are easy for humans, such as controlling a video game and solving a visual puzzle, but particularly difficult for these models.
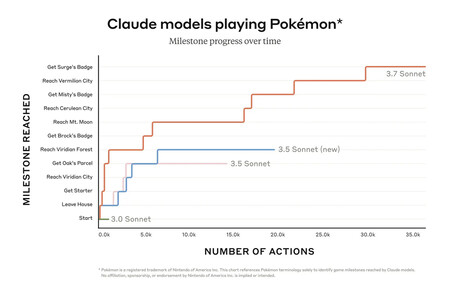
Anthropic’s progress is remarkable but far from a success. According to Ars Technica, thousands of viewers on the Twitch channel created by Anthropic have watched Claude get stuck on Mount Sélénite, one of the game’s sections.
On this channel, viewers can also see Claude repeatedly trying to solve the problem and move forward. It “thinks” and “reasons,” even displaying its thought process, but the model still struggles to progress through this phase of the game.
Yet This Represents a Significant Achievement for AI Models
Considering that the game is designed for children, it may be easy to dismiss Anthropic’s accomplishment. However, these advances should be viewed positively. First, the Claude 3.7 model used wasn’t pre-trained to play, it had to learn and adapt on the fly.
Claude “sees” the screen, analyzes the gameplay, and reacts accordingly. The challenge lies in the game’s simple, pixelated graphics, which make interpretation difficult for the model. With better graphics, Claude would likely perform much better, according to one of the experimenters.
However, Claude recognizes the necessary actions in sections of the game where text is displayed, allowing it to excel in those stages.
A serious limitation is its ability to memorize. Claude struggles to retain everything it has learned, as it has a limited memory of 200,000 tokens. When the AI model reaches this limit, it summarizes and condenses information, sometimes eliminating small details that are crucial for progress.
Despite these challenges, Anthropic’s performance remains remarkable and suggests a future where AI models will play autonomously and excel in all types of games. Just as DeepMind succeeded with Breakout, AI models could achieve similar feats on a much larger scale.
Images | Anthropic
Log in to leave a comment