The definition of open source has been clear for years. The definition of open source AI isn’t. This lack of consensus about whether an AI model is genuinely open source has sparked controversy, with many claiming their models are open source when they probably aren’t. Meta is a prime example of this situation.
This is exactly what the Open Source Initiative (OSI), responsible for the original definition of open source, is now trying to address as it works to create a universal, standard definition of open source AI.
The effort seems to be making progress. The OSI recently announced the release of the first candidate version (RC1) of that definition. It specifies four fundamental aspects that an AI system must have to be considered open source:
- It can be used for any purpose without permission.
- It can be studied to analyze how it works.
- It can be modified for any purpose.
- It can be redistributed with or without modifications.
Purists vs. Companies
However, this proposed definition includes certain elements that have sparked debate between purists and advocates of a more relaxed standard.
The OSI has been willing to compromise slightly on the issue of training data. It recognizes that it’s difficult for companies to share the full details of the datasets they’ve used to train their models. As a result, RC1 requires “sufficiently detailed information about the data used to train the system” rather than full dataset disclosure. This initiative aims to balance transparency with legal and practical considerations.
For purists, however, this isn’t enough. If the AI system doesn’t provide fully open data about the information it uses, they argue that LLMs cannot be considered open source.
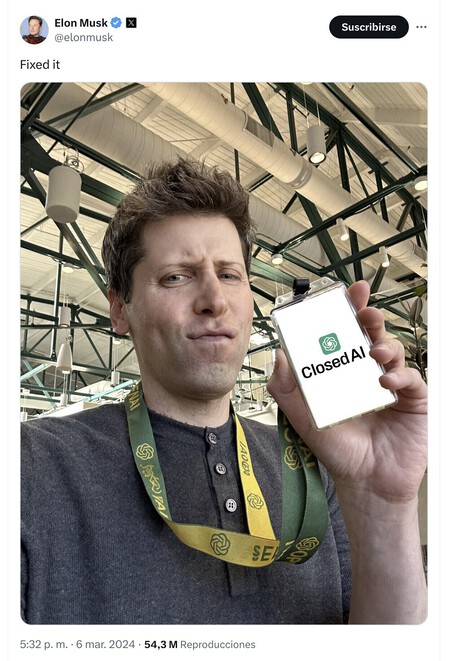
The OSI has a strong argument: If AI systems are required to disclose all their information, it would “relegate open source AI to a niche... because the amount of freely and legally shareable data is a tiny fraction of what is necessary to train powerful systems.”
Total Transparency Is Utopian
As OSI director Stefano Maffulli explained, training data falls into four categories: open, public, obtainable, and unshareable. According to OSI, “the legal requirements are different for each. All are required to be shared in the form that the law allows them to be shared.” OSI’s reasoning is logical—sharing data, or at least part of it, is challenging.
In an interview with ZDNet, Maffulli noted that open source purists aren’t the only ones making the definition of open source AI difficult.
On the other extreme are companies that consider their training methods, data collection, filtering processes, and dataset creation to be trade secrets. Asking these companies to reveal this information is similar to asking Microsoft to reveal the source code of Windows in the 1990s.
The OSI has introduced two notable changes from previous drafts in this proposed definition. First, the model must provide enough information to understand how companies conducted the training. This makes it possible to, among other things, to create variations, or “forks,” of AI systems.
Second, creators can explicitly impose copyleft conditions (to allow free use and redistribution of work) for the open source AI’s code, data, and parameters. For example, this would make it possible to require a copyleft license that binds the training code to the dataset used to train the model.
The release of this first candidate version of the definition is undoubtedly an essential step toward consensus on this unique issue. However, there’s still work to be done. The OSI will reportedly announce the final 1.0 version of the open source AI definition at the All Things Open conference on Oct. 28. And even then, it will be just the first version.
Image | Meta Connect 2024
View 0 comments