OpenAI discussed the two scaling laws for neural language models in a study from 2020. Analyst Ethan Mollick recently analyzed what they mean for the models currently dominating the AI industry.
The bigger, the better. The first scaling law refers to training and says that larger models offer more features. However, training these models requires increasingly substantial amounts of computation, data, and energy. This explains why companies are investing significant resources to “scale” AI models. They’re pouring in vast sums of money even though recent improvements in capacity have been less impressive than in the past.
The more they think, the better they are. Surprisingly, the second scaling law suggests that AI models can perform better if they’re given more time to think. According to Mollick, OpenAI discovered with model o1 that allowing a model to spend additional time and resources on inference leads to improved results when solving problems and answering questions. This has sparked a trend toward developing reasoning models.
Massive AI models. By combining these two laws, AI companies are now developing models that are larger and more capable than ever. However, the advancements aren’t as impressive as those of previous generations. Companies are improving reasoning skills to bridge this gap, allowing AI models to deliver much more accurate answers. Although these models aren’t flawless, the combination of both scaling laws is proving effective.
Large models can lead to the creation of impressive smaller models. DeepSeek R1 has demonstrated this: Large models can be the foundation for smaller and much cheaper models that perform exceptionally well. If these models also possess reasoning capabilities, this combination is even more promising.
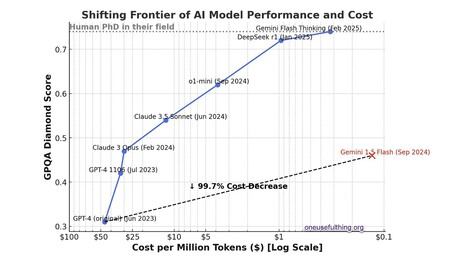
AI technology is continually improving and becoming more affordable. Experts have long pointed out the ongoing decline in the cost of using AI models. When OpenAI introduced GPT-4, the price was $50 per million tokens. With the arrival of Gemini 1.5 Flash, which surpasses GPT-4 in performance, that cost has plummeted to just $0.12 per million tokens, a remarkable 99.7% reduction.
All this investment is worthwhile. Many AI companies are currently burning through millions of dollars, but they understand that this isn’t the time to focus on profitability. They’re aware that the future holds the potential for improved models at a lower price point. This is the phase of heavy investment rather than profitability, aiming to avoid future costs.
AI won’t only enhance productivity. Mollick suggests that people should change their perspective on AI and stop viewing it solely as a means to automate routine, low-level jobs. “We will need to consider the ways in which AI can serve as a genuine intellectual partner,” he says. As such, AI models can help create knowledge and make discoveries that no one may have considered or would have taken considerably longer to uncover.
Image | Saradasish Pradhan
Related | What Are Distilled AI Models? A Look at LLM Distillation and Its Outputs
Log in to leave a comment